How to read and interpret snRNAseq results
Introduction to snRNAseq: Understanding the basics
snRNAseq, or single nucleus RNA sequencing, is an innovative technology that enables analysis of gene expression in single cells. Unlike traditional sequencing methods, snRNAseq allows us to understand cellular heterogeneity in tissues, which is crucial for many biological and medical studies.
As more and more studies use this advanced method, it is important for researchers and students to know how to not only interpret, but also correctly read snRNAseq results. In this article, we will discuss the main steps involved in analyzing and interpreting this data.
Preparing for snRNAseq analysis
Before analyzing snRNAseq data, it is important to pay attention to several important aspects. First, it is important to thoroughly understand sample preparation protocols to avoid potential errors that can distort the results.
In the sample preparation process, steps such as cell isolation, cell lysis and RNA cloning are particularly important. A properly performed isolation process is key to obtaining high-quality results that can later be analyzed without the risk of duplicating errors.
Choice of bioinformatics tools
Analysis of snRNAseq data requires the use of appropriate bioinformatics tools. There are many software and packages that can be used to analyze such data. The most popular are "Seurat", "Scanpy" and "Monocle", which offer different functionalities that allow you to choose the appropriate approach for analysis.
The choice of tools should depend on the specifics of the study and the purpose of the analysis. For example, if the goal is to identify subpopulations of cells, the Seurat package may be the best choice due to its advanced clustering algorithms.
Data analysis: Processing steps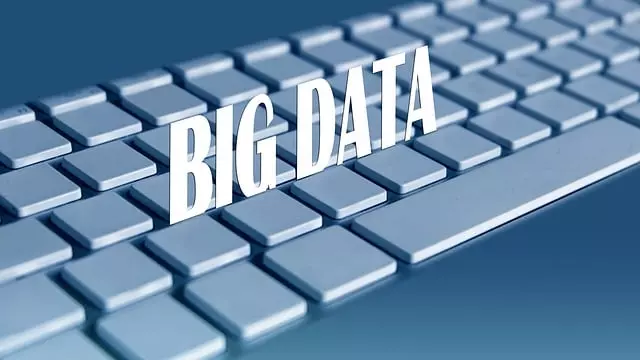
Analysis of snRNAseq data can be divided into several key stages. Each requires a detailed approach and understanding. These are:
Poor data quality: The first step is to assess the quality of the data obtained. It is worth checking the samples for genomic contamination, which can affect the analysis results.Data normalization: Normalization is a key step to compare data from different cells. Techniques such as scaling and log-transformation are commonly used here.Clustering: This step groups cells based on their gene expression profile. It is useful to use different clustering methods and compare the results to get the most reliable data.Differential expression analysis: After clusterization, you can proceed to differential gene expression analysis to identify which genes are differentially expressed in different cell groups.Interpretation of snRNAseq results
Once the data analysis is complete, the interpretation of the data must be addressed. It is important to understand what implications the results have for the broader biological context. The snRNAseq results can provide information about biological dynamics in tissues, cellular match, and potential therapeutic targets.
Also, it is important to bring out important gene expression differences that may have clinical relevance. Proper interpretation of the results can lead to discoveries in disease pathophysiology and provide information on therapeutic options.
Summary and future of snRNAseq
snRNAseq is a powerful tool that deserves in-depth analysis and interpretation. With each passing year, the technology is becoming more accessible, making its importance in biological and medical research only growing. Developments in analytical algorithms and sequencing techniques are contributing to the efficiency of snRNAseq data analysis.
For the future, we can expect more and more new discoveries that will help us better understand the complexity of life at the cellular level. Ultimately, the ability to focus on accurate interpretation of results will become a key element for researchers around the world.
Add comment